Particle filters and curse of dimensionality
Duration: 37 mins 11 secs
Share this media item:
Embed this media item:
Embed this media item:
About this item
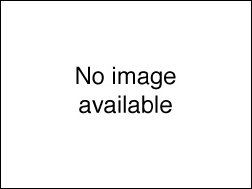
Description: |
Rebeschini, P (Princeton University)
Thursday 24 April 2014, 11:40-12:15 |
---|
Created: | 2014-04-28 17:29 |
---|---|
Collection: | Advanced Monte Carlo Methods for Complex Inference Problems |
Publisher: | Isaac Newton Institute |
Copyright: | Rebeschini, P |
Language: | eng (English) |
Distribution: |
World
![]() |
Explicit content: | No |
Aspect Ratio: | 16:9 |
Screencast: | No |
Bumper: | UCS Default |
Trailer: | UCS Default |
Abstract: | Co-author: Ramon van Handel (Princeton University)
A problem that arises in many applications is to compute the conditional distributions of stochastic models given observed data. While exact computations are rarely possible, particle filtering algorithms have proved to be very useful for approximating such conditional distributions. Unfortunately, the approximation error of particle filters grows exponentially with dimension, a phenomenon known as curse of dimensionality. This fact has rendered particle filters of limited use in complex data assimilation problems that arise, for example, in weather forecasting. In this talk I will argue that it is possible to develop “local” particle filtering algorithms whose approximation error is dimension-free. By exploiting conditional decay of correlations properties of high-dimensional models, we prove for the simplest possible algorithm of this type an error bound that is uniform both in time and in the model dimension. (Joint work with R. van Handel) Related Links: http://arxiv.org/abs/1301.6585 - Preprint |
---|
Available Formats
Format | Quality | Bitrate | Size | |||
---|---|---|---|---|---|---|
MPEG-4 Video | 640x360 | 1.94 Mbits/sec | 541.35 MB | View | Download | |
WebM | 640x360 | 492.67 kbits/sec | 134.23 MB | View | Download | |
iPod Video | 480x270 | 522.12 kbits/sec | 142.19 MB | View | Download | |
MP3 | 44100 Hz | 249.8 kbits/sec | 68.09 MB | Listen | Download | |
Auto * | (Allows browser to choose a format it supports) |