On the uniform ergodicity of the particle Gibbs sampler
Duration: 41 mins 20 secs
Share this media item:
Embed this media item:
Embed this media item:
About this item
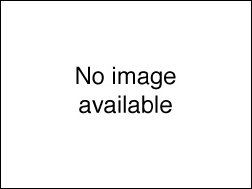
Description: |
Moulines, E (Télécom ParisTech)
Tuesday 22 April 2014, 11:05-11:40 |
---|
Created: | 2014-04-28 17:24 |
---|---|
Collection: | Advanced Monte Carlo Methods for Complex Inference Problems |
Publisher: | Isaac Newton Institute |
Copyright: | Moulines, E |
Language: | eng (English) |
Distribution: |
World
![]() |
Explicit content: | No |
Aspect Ratio: | 16:9 |
Screencast: | No |
Bumper: | UCS Default |
Trailer: | UCS Default |
Abstract: | Co-authors: Randal Douc (Telecom SudParis), Fred Lindsten (Cambridge)
The particle Gibbs sampler is a systematic way of using a particle filter within Markov chain Monte Carlo (MCMC). This results in an off-the-shelf Markov kernel on the space of state trajectories, which can be used to simulate from the full joint smoothing distribution for a state space model in an MCMC scheme. We show that the PG Markov kernel is uniformly ergodic under rather general assumptions, that we will carefully review and discuss. In particular, we provide an explicit rate of convergence which reveals that: (i) for fixed number of data points, the convergence rate can be made arbitrarily good by increasing the number of particles, and (ii) under general mixing assumptions, the convergence rate can be kept constant by increasing the number of particles superlinearly with the number of observations. We illustrate the applicability of our result by studying in detail two common state space models with non-compact state spaces. |
---|
Available Formats
Format | Quality | Bitrate | Size | |||
---|---|---|---|---|---|---|
MPEG-4 Video | 640x360 | 1.94 Mbits/sec | 601.65 MB | View | Download | |
WebM | 640x360 | 479.65 kbits/sec | 145.27 MB | View | Download | |
iPod Video | 480x270 | 522.12 kbits/sec | 158.06 MB | View | Download | |
MP3 | 44100 Hz | 249.82 kbits/sec | 75.69 MB | Listen | Download | |
Auto * | (Allows browser to choose a format it supports) |