Consistency and CLTs for stochastic gradient Langevin dynamics based on subsampled data
Duration: 21 mins 32 secs
Share this media item:
Embed this media item:
Embed this media item:
About this item
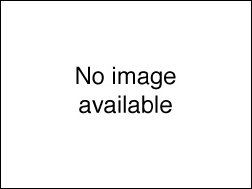
Description: |
Vollmer, S (University of Oxford)
Thursday 24 April 2014, 15:50-16:25 |
---|
Created: | 2014-04-25 13:48 |
---|---|
Collection: | Advanced Monte Carlo Methods for Complex Inference Problems |
Publisher: | Isaac Newton Institute |
Copyright: | Vollmer, S |
Language: | eng (English) |
Distribution: |
World
![]() |
Explicit content: | No |
Aspect Ratio: | 16:9 |
Screencast: | No |
Bumper: | UCS Default |
Trailer: | UCS Default |
Abstract: | Co-authors: Alexandre Thiery (National University of Singapore), Yee-Whye Teh (University of Oxford)
Applying MCMC to large data sets is expensive. Both calculating the acceptance probability and creating informed proposals depending on the likelihood require an iteration through the whole data set. The recently proposed Stochastic Gradient Langevin Dynamics (SGLD) circumvents this problem by generating proposals based on only a subset of the data and skipping the accept-reject step. In order to heuristically justify the latter, the step size converges to zero in a non-summable way. Under appropriate Lyapunov conditions, we provide a rigorous foundation for this algorithm by showing consistency of the weighted sample average and proving a CLT for it. Surprisingly, the fraction of the data subset selection does not have an influence on the asymptotic variance. |
---|
Available Formats
Format | Quality | Bitrate | Size | |||
---|---|---|---|---|---|---|
MPEG-4 Video | 640x360 | 1.94 Mbits/sec | 313.52 MB | View | Download | |
WebM | 640x360 | 463.93 kbits/sec | 73.23 MB | View | Download | |
iPod Video | 480x270 | 522.14 kbits/sec | 82.35 MB | View | Download | |
MP3 | 44100 Hz | 249.73 kbits/sec | 39.45 MB | Listen | Download | |
Auto * | (Allows browser to choose a format it supports) |