Decision-making under uncertainty: Using subjective probabilistic judgements for decision support in pollinator abundance and food security
Duration: 17 mins 57 secs
Share this media item:
Embed this media item:
Embed this media item:
About this item
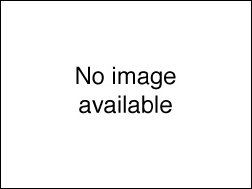
Description: |
Barons, M
Thursday 4th July 2019 - 12:10 to 12:30 |
---|
Created: | 2019-07-05 08:39 |
---|---|
Collection: | Current status and key questions in Landscape Decision making |
Publisher: | Isaac Newton Institute |
Copyright: | Barons, M |
Language: | eng (English) |
Distribution: |
World
![]() |
Explicit content: | No |
Aspect Ratio: | 16:9 |
Screencast: | No |
Bumper: | UCS Default |
Trailer: | UCS Default |
Abstract: | Hunger and food poverty is on the increase even in developed nations like the UK, USA, Canada & Australia. With a growing population and a finite planet, there is urgent need for action, but in such a large, complex system identifying the most effective action requires decision support.
Food security exists when all people, at all times, have physical and economic access to sufficient, safe and nutritious food to meet their dietary needs and food preferences for an active and healthy life. In order to provide decision support, it is necessary to elicit probability distributions to evaluate subjective expected utility scores associated with ameliorating policies that might be enacted. When the underlying process model is extremely large and complex, this brings its own peculiar challenges. It is first necessary to elicit the overall, agreed structure describing in broad terms the underlying nature of the system from representatives of all domain experts across the system as a whole. We have now shown that this can be done formally and consistently with probability models if the elicitations concern the elicitation of dependences – formally termed irrelevances (Smith, Barons and Leonelli (2016)). Within a probability model, these irrelevance statements then transform into assertions about various conditional independence statements. These, in turn, can be used to determine how the system can be divided up into (conditionally) independent segments. The quantitative expert judgements associated with each segment of the process can then be delegated to a relevant panel of experts. The implicit (albeit virtual) owner of beliefs expressed in the system will be referred to as the supraBayesian , meaning that the decision-making group acts as a single person would and it is her coherence that we are concerned with. Under suitable conditions it can then be shown that the elicited overarching structure can compose these judgments together to form a coherent probabilistic model to score different options available to the user, termed an integrating decisions support system (IDSS). One element of the overarching food poverty models is food supply, and key to parts of this is an abundant and healthy population of pollinating insects to pollination services for food. In 2014 the UK government undertook a consultation and produced their pollinator strategy for the next 10 years “to see pollinators thrive, providing essential pollination services and benefits for food production, the wider environment and everyone.” However, the evidence base on the complex system driving pollinator vigour and numbers is patchy and held in disparate domains of expertise, making the evaluation of policy options problematic. In this talk I will describe how we are in the process of developing an IDSS based on these theoretical developments, and how a probabilistic model for pollinator abundance incorporating structured expert elicitation will then form a sub-module of this IDSS for policies relating to household food insecurity. J. Q. Smith, M.J. Barons, and M. Leonelli. Coherent inference for integrating decision support systems. arXiv, 2016. http://arxiv.org/abs/1507.07394. Co-authors: Jim Q. Smith, Manuele Leonelli |
---|
Available Formats
Format | Quality | Bitrate | Size | |||
---|---|---|---|---|---|---|
MPEG-4 Video | 640x360 | 1.94 Mbits/sec | 261.35 MB | View | Download | |
WebM | 640x360 | 714.3 kbits/sec | 94.00 MB | View | Download | |
iPod Video | 480x270 | 522.18 kbits/sec | 68.65 MB | View | Download | |
MP3 | 44100 Hz | 249.87 kbits/sec | 32.88 MB | Listen | Download | |
Auto * | (Allows browser to choose a format it supports) |