Scalable algorithms for Markov process parameter inference
48 mins 16 secs,
307.42 MB,
WebM
640x360,
29.97 fps,
44100 Hz,
869.6 kbits/sec
Share this media item:
Embed this media item:
Embed this media item:
About this item
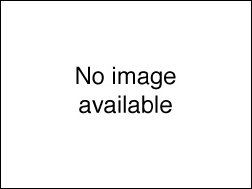
Description: |
Wilkinson, D (Newcastle University)
Friday 8th April 2016 - 09:45 to 10:30 |
---|
Created: | 2016-04-12 10:39 |
---|---|
Collection: | Stochastic Dynamical Systems in Biology: Numerical Methods and Applications |
Publisher: | Isaac Newton Institute |
Copyright: | Wilkinson, D |
Language: | eng (English) |
Distribution: |
World
![]() |
Explicit content: | No |
Aspect Ratio: | 16:9 |
Screencast: | No |
Bumper: | UCS Default |
Trailer: | UCS Default |
Abstract: | Inferring the parameters of continuous-time Markov process models using partial discrete-time observations is an important practical problem in many fields of scientific research. Such models are very often "intractable", in the sense that the transition kernel of the process cannot be described in closed form, and is difficult to approximate well. Nevertheless, it is often possible to forward simulate realisations of trajectories of the process using stochastic simulation. There have been a number of recent developments in the literature relevant to the parameter estimation problem, involving a mixture of approximate, sequential and Markov chain Monte Carlo methods. This talk will compare some of the different "likelihood free" algorithms that have been proposed, including sequential ABC and particle marginal Metropolis Hastings, paying particular attention to how well they scale with model complexity. Emphasis will be placed on the problem of Bayesian pa rameter inference for the rate constants of stochastic biochemical network models, using noisy, partial high-resolution time course data. |
---|
Available Formats
Format | Quality | Bitrate | Size | |||
---|---|---|---|---|---|---|
MPEG-4 Video | 640x360 | 1.94 Mbits/sec | 702.81 MB | View | Download | |
WebM * | 640x360 | 869.6 kbits/sec | 307.42 MB | View | Download | |
iPod Video | 480x270 | 522.15 kbits/sec | 184.53 MB | View | Download | |
MP3 | 44100 Hz | 249.76 kbits/sec | 88.36 MB | Listen | Download | |
Auto | (Allows browser to choose a format it supports) |