Linking stochastic dynamic biological models to data: Bayesian inference for parameters and structure
48 mins,
305.34 MB,
WebM
640x360,
29.97 fps,
44100 Hz,
868.52 kbits/sec
Share this media item:
Embed this media item:
Embed this media item:
About this item
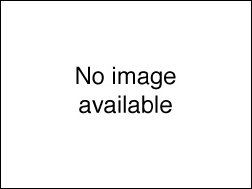
Description: |
Wilkinson, D (Newcastle University)
Tuesday 19th January 2016 - 11:45 to 12:30 |
---|
Created: | 2016-01-25 16:00 |
---|---|
Collection: | Stochastic Dynamical Systems in Biology: Numerical Methods and Applications |
Publisher: | Isaac Newton Institute |
Copyright: | Wilkinson, D |
Language: | eng (English) |
Distribution: |
World
![]() |
Explicit content: | No |
Aspect Ratio: | 16:9 |
Screencast: | No |
Bumper: | UCS Default |
Trailer: | UCS Default |
Abstract: | Within the field of systems biology there is increasing interest in developing computational models which simulate the dynamics of intra-cellular biochemical reaction networks and incorporate the stochasticity inherent in such processes. These models can often be represented as nonlinear multivariate Markov processes. Analysing such models, comparing competing models and fitting model parameters to experimental data are all challenging problems. This talk will provide an overview of a Bayesian approach to the problem. Since the models are typically intractable, use is often made of algorithms exploiting forward simulation from the model in order to render the analysis "likelihood free". There have been a number of recent developments in the literature relevant to this problem, involving a mixture of sequential and Markov chain Monte Carlo methods. Particular emphasis will be placed on the problem of Bayesian parameter inference for the rate constants of stochastic b iochemical network models, using noisy, partial high-resolution time course data, such as that obtained from single-cell fluorescence microscopy studies. |
---|
Available Formats
Format | Quality | Bitrate | Size | |||
---|---|---|---|---|---|---|
MPEG-4 Video | 640x360 | 1.94 Mbits/sec | 698.56 MB | View | Download | |
WebM * | 640x360 | 868.52 kbits/sec | 305.34 MB | View | Download | |
iPod Video | 480x270 | 522.2 kbits/sec | 183.52 MB | View | Download | |
MP3 | 44100 Hz | 249.75 kbits/sec | 87.86 MB | Listen | Download | |
Auto | (Allows browser to choose a format it supports) |